RexLegendi started reading De opwindvogelkronieken by Haruki Murakami
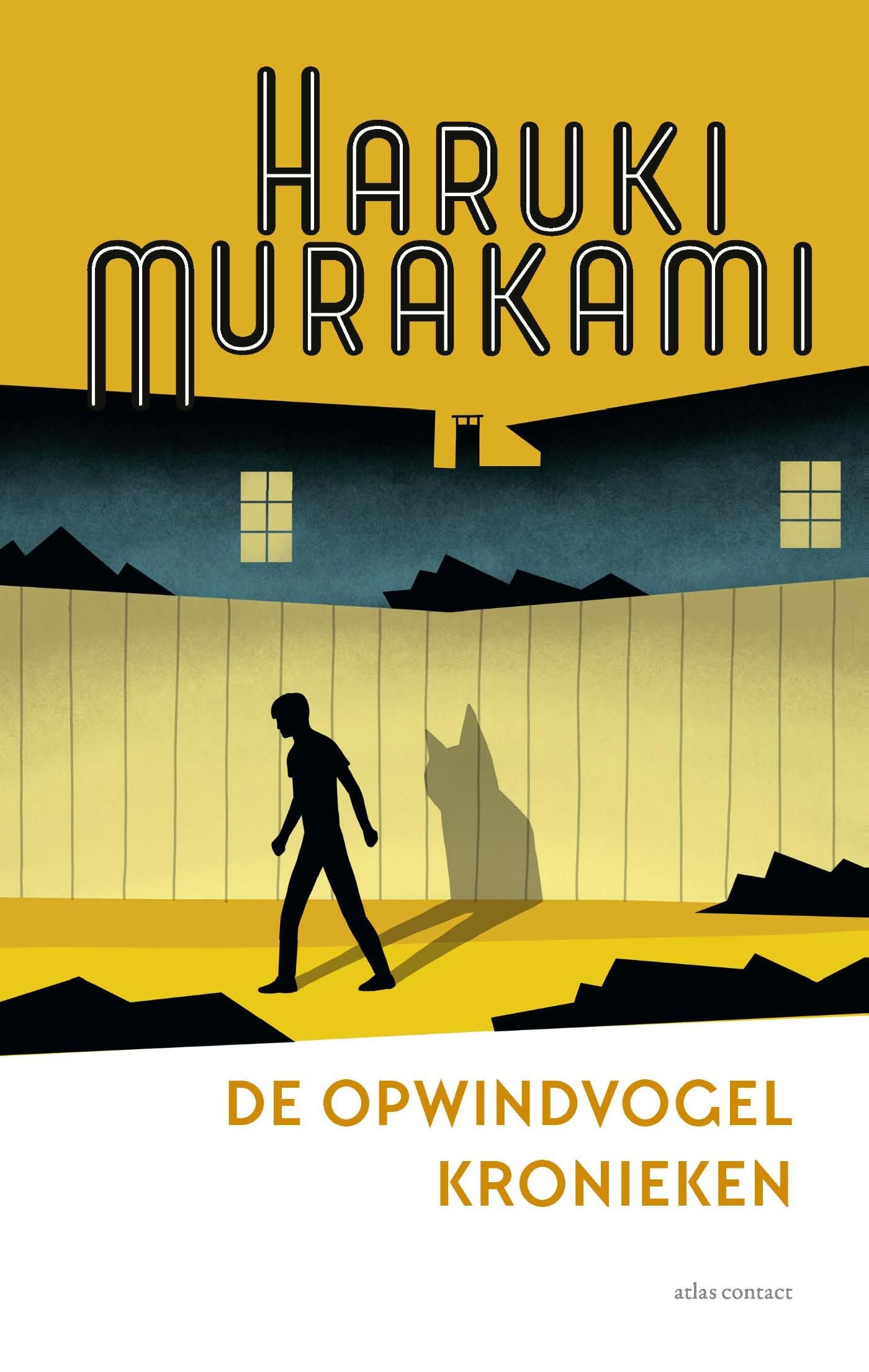
De opwindvogelkronieken by Haruki Murakami
In De opwindvogelkronieken van Haruki Murakami slaat het noodlot wel erg hard toe bij Tōru Okada: hij raakt zijn baan …
Dutch Parisian, thirtysomething, fanatic reader ever since I regained time. I write reviews for my own memory and critical development, but since they are public, I try to do so in a way others may appreciate them too. (I certainly enjoy reading other people's reviews, so thank you for writing them!)
Comparing books is like comparing apples and oranges, therefore stars are categories rather than rankings:
★ 😡 (Waste of time) ★★ 👎🏼 (Not my thing) ★★★ 👍🏼 (Worth reading) ★★★★ 👏🏼 (Highly recommended) ★★★★★ ❤️ (Coup de cœur)
This link opens in a pop-up window
37% complete! RexLegendi has read 29 of 78 books.
In De opwindvogelkronieken van Haruki Murakami slaat het noodlot wel erg hard toe bij Tōru Okada: hij raakt zijn baan …
Thousand Cranes was my first encounter with Nobel Prize laureate Yasunari Kawabata (1899-1972). It is an intimate portrait of a young man, Kikuji Mitani, an orphan and bachelor, navigating the contrasting influences of his late father’s mistresses, Chikako and Mrs. Ota. Too inexperienced to suspect their true intentions, Kikuji initially goes with the flow, attending Chikako’s tea ceremony to meet a young lady and ending up in bed with Mrs. Ota. There is an interesting passage about the birthmark on Chikako’s breast, which raises questions about her value as a woman. At first described as ‘sexless’ and ‘turned masculine,’ Chikako gradually reveals herself to be rather cunning, attempting to manipulate Kikuji into marriage.
The novel felt both traditional and modern at the same time. One the one hand, I recognised some of the themes I encountered in Jun'ichirō Tanizaki’s The Makioka Sisters, such as the unspoken code between people …
Thousand Cranes was my first encounter with Nobel Prize laureate Yasunari Kawabata (1899-1972). It is an intimate portrait of a young man, Kikuji Mitani, an orphan and bachelor, navigating the contrasting influences of his late father’s mistresses, Chikako and Mrs. Ota. Too inexperienced to suspect their true intentions, Kikuji initially goes with the flow, attending Chikako’s tea ceremony to meet a young lady and ending up in bed with Mrs. Ota. There is an interesting passage about the birthmark on Chikako’s breast, which raises questions about her value as a woman. At first described as ‘sexless’ and ‘turned masculine,’ Chikako gradually reveals herself to be rather cunning, attempting to manipulate Kikuji into marriage.
The novel felt both traditional and modern at the same time. One the one hand, I recognised some of the themes I encountered in Jun'ichirō Tanizaki’s The Makioka Sisters, such as the unspoken code between people – particularly between men and women – the tea rituals, and the intended ‘miai’. On the other hand, there is a level of intimacy and openness I couldn’t quite place. I’m looking forward to reading The Sound of the Mountain and Beauty and Sadness.
Er zijn twee uitstekende redenen om Voor ieder wat waars (2023) van Rob Wijnberg te lezen. Ten eerste is de filosoof en hoofdredacteur van De Correspondent een uiterst intelligente en gestructureerde denker, die helder inzicht biedt in de staat van onze samenleving. Ten tweede begrijpt Wijnberg waar mensen behoefte aan hebben: een vergezicht, hoop in tijden waarin eenieder zijn eigen waarheid lijkt na te streven terwijl de planeet op ontploffen staat. De schrijver waagt zich aan een nieuw perspectief, gericht op ecologisme, een duurzame revolutie en collectieve voorspoed. Daarin is hij misschien niet uniek, maar de manier waarop hij eerst uiteenzet hoe de betekenis van waarheid door de menselijke geschiedenis heen is veranderd en vervolgens hoe de media en politiek die betekenis drastisch hebben beïnvloed, biedt een waardevolle context voor die boodschap.
De post-truth society bestaat niet In zijn inleiding beschrijft Wijnberg hoe ons begrip van de ‘waarheid’ bepaalt hoe …
Er zijn twee uitstekende redenen om Voor ieder wat waars (2023) van Rob Wijnberg te lezen. Ten eerste is de filosoof en hoofdredacteur van De Correspondent een uiterst intelligente en gestructureerde denker, die helder inzicht biedt in de staat van onze samenleving. Ten tweede begrijpt Wijnberg waar mensen behoefte aan hebben: een vergezicht, hoop in tijden waarin eenieder zijn eigen waarheid lijkt na te streven terwijl de planeet op ontploffen staat. De schrijver waagt zich aan een nieuw perspectief, gericht op ecologisme, een duurzame revolutie en collectieve voorspoed. Daarin is hij misschien niet uniek, maar de manier waarop hij eerst uiteenzet hoe de betekenis van waarheid door de menselijke geschiedenis heen is veranderd en vervolgens hoe de media en politiek die betekenis drastisch hebben beïnvloed, biedt een waardevolle context voor die boodschap.
De post-truth society bestaat niet In zijn inleiding beschrijft Wijnberg hoe ons begrip van de ‘waarheid’ bepaalt hoe we naar de werkelijkheid kijken en wat we wel en niet zien. Die waarheid bevat bovendien een belofte: ze geeft richting en zin aan het bestaan. In onze samenleving – het betoog lijkt zich te beperken tot het Westen – was die belofte lange tijd gericht op vooruitgang, vormgegeven door het liberalisme (rechtsstaat, vrije markt en vrijheid van het individu) en de sociaaldemocratie. Deze belofte heeft echter aan verbeeldingskracht verloren als gevolg van een paradox. Aan de ene kant hebben mensen in westerse samenlevingen het nog nooit zo goed gehad; we zijn zelfs zo gezond, veilig, welvarend en geïnformeerd dat men over het ‘antropoceen’ spreekt. Aan de andere kant kampen we met de grootste problemen uit de menselijke geschiedenis: dezelfde fossiele grondstoffen waaraan we alles te danken hebben, ruïneren ons nu. Bij gebrek aan een samenhangend verhaal is het ‘nostalgisch nationalisme’ opgekomen. Kenmerkend zijn het ontkennen van de paradox, het zoeken naar vooruitgang in het verleden en de belofte van een terugkeer naar een zorgeloze tijd. Gebaat bij het ontbreken van een eenduidige waarheid, zaait het twijfel over vertrouwde bronnen: pers, rechtspraak en wetenschap. Wijnberg verzet zich echter tegen de idee van een ‘post-truth society’, die veronderstelt dat er niet langer een gedeelde waarheid bestaat. In plaats daarvan spreekt hij liever over een ‘post-progress society’, verbolgen over het verbreken van de belofte. Die biedt hoop voor het vinden van een oplossing.
Constateren dat de waarheid haar zeggingskracht is verloren, kan alleen als er nog een notie van waarheid bestaat. Je kunt namelijk niet spreken van nepnieuws zonder het verschil te zien met waarachtig nieuws. Je kunt niet spreken van feitenvrije politiek zonder te weten welke feiten dan worden weggelaten of verdraaid. Je kunt niet spreken van leugens zonder een idee te hebben van wat wél eerlijk was geweest. Je kunt, kortom, niet spreken van een samenleving voorbij de waarheid zonder een waarheid te veronderstellen.
Waarheid als verlossing Centraal in het betoog staat de betekenis van waarheid. Om grip te krijgen op de huidige problemen, onderzoekt Wijnberg hoe die zich door de eeuwen heen heeft ontwikkeld. Daarbij onderscheidt hij vier perioden in de menselijke (westerse) geschiedenis: de premoderne tijd (400 v.Chr. - 1600), de moderne tijd (1600-1950), de postmoderne tijd (vanaf 1950) en als verbijzondering daarvan de postmoderne consumptietijd (vanaf 1980). De vergelijking tussen heden en verleden is nuttig, omdat ze duidelijk maakt dat onze notie van ‘waarheid’ veranderlijk is. Zo begint Wijnberg met de premoderne mens voor wie – in lijn met Plato – er twee werkelijkheden bestonden: één zoals die ‘is’ en één zoals die aan ons ‘verschijnt’. Ook in de grote religies is een dergelijk onderscheid (‘hemel’ en ‘aarde’) vindbaar. Om deze notie te verklaren, is van belang dat de premoderne mens natuurverschijnselen nog niet kon verklaren zoals dat nu gebeurt en het leven zwaar was; bij gebrek aan maatschappelijke mobiliteit gold de waarheid (het ‘transcendente’) als een verlossing voor het leven op aarde.
Ontdekking van de tijd In de moderne tijd zoekt de mens niet langer naar waarheid in het bovenaardse, maar in de wereld om hem heen: in de term ‘objectiviteit’ weerklinkt de waarheid als weerspiegeling van de werkelijkheid. De mens construeert niet alleen technologie, maar ook mensenrechten, de natiestaat en de idee van tijd als lineaire reis. Weliswaar is het leven nog zwaar, maar dankzij een geloof in vooruitgang niet langer uitzichtloos. Dat dezelfde opvatting een keerzijde heeft, blijkt wanneer ze in de twintigste eeuw tot excessen leidt.
Bevrijding van de individu Na de Tweede Wereldoorlog raakt de idee in zwang dat de mens de waarheid zelf voortbrengt: ze is dus niet ‘gegeven’ of ‘gevonden’, maar ‘gemaakt’. De opvatting dat de geschiedenis zich naar een bepaald eindpunt beweegt, maakt plaats voor nieuwe ideeën over existentialisme en identiteitspolitiek, terwijl het neoliberalisme de individu tracht te bevrijden van een laatste obstakel: het gemeenschapsdenken. Tussen economische zelfstandigheid en de ontplooiing van de individu is in de vrijemarktsamenleving geen plaats voor een collectief of moreel karakter.
Consumeren van waarheid Als gevolg van de nadruk op de vrije markt verschuift de aandacht van het bevrijden van de individu naar het bevredigen van behoeften: in de postmoderne consumptietijd maken we geen waarheid, maar consumeren we die. Wijnberg is op zijn scherpst wanneer hij de huidige ontwikkelingen in de media en politiek duidt. Informatie is steeds vaker ‘content’, dat wil zeggen inhoud + tevredenheid, afgestemd op de doelgroep. Mede dankzij algoritmen ontstaat een geprivatiseerde ruimte voor informatie ‘die bij ons past’, ten koste van een gemeenschappelijke realiteit.
Waar is niet wat klopt. Waar is wat klikt. […] Zo kijken we door het grootste raam op de wereld dat de mens ooit heeft uitgevonden, kunnen we verder de wereld in kijken dan ooit, en zien we: onszelf.
Cynisme Het nieuws verandert wezenlijk. Zowel traditionele als sociale media bouwen voort op de nieuwe betekenis van waarheid als consumptiegoed. Interessant is bijvoorbeeld hoe de algoritmen van online platformen zijn afgestemd op ophef: meer is beter. Een platform als X is daarom niet zozeer gevaarlijk omdat het sociale bubbels zou creëren – Wijnberg stelt dat de vroegere zuilen erger waren – maar omdat het als vergrootglas werkt: 91% van de tweets (en 97% van de politieke tweets) is geproduceerd door gebruikers die uiterst links of rechts van het spectrum staan. Ondertussen denderen de ‘actualiteiten’ door als een onophoudelijk schallende sirene. Veel media voorzien complexe problemen van eendimensionale verklaringen die vooral tonen hoe de wereld niet werkt. Het schadelijke gevolg is dat nieuws vooral verontwaardiging wekt en tot een ongezond wantrouwen en cynisme (‘iedereen is corrupt’) leidt. Dat diezelfde media aan ‘geïnstitutionaliseerd geheugenverlies’ leiden, verergert het beeld.
Ziedaar de moderne nieuwsvoorziening in een notendop. Nieuws als permanente verontwaardiging zonder richtingsgevoel: alles een schande vinden, maar nergens de samenhang in zien. Voortdurend het vertrouwen in mensen als naïef en goedgelovig afschilderen om vervolgens schande te spreken van het zwartgallige mensbeeld dat daarmee wordt gekweekt.
Dat ook kwaliteitsmedia cynisch kunnen zijn, laat Wijnberg nogmaals zien wanneer hij het werk van zijn collega Jesse Frederik ([book:Zo hadden we het niet bedoeld|56049058]) aanhaalt. Omdat twijfel die uit cynisme voortkomt niet gebaseerd is op inhoudelijke gronden, maar op argwaan over motieven, ontstaat een samenspel van journalistiek wantrouwen, een verdienmodel dat drijft op het genereren van aandacht en politici die hun bestaansrecht ontlenen aan zichtbaarheid.
Toekomst: de verbonden tijd In context bezien is deze diagnose een stuk duidelijker. Om uit de houdgreep van de huidige waarheidsopvatting te komen, is het nodig de kaders te herijken. Zolang we vooruitgang uitsluitend zien in termen van technologie, kennis en moraal, beperken we ons tot de kaders van de (post)moderne tijd. Om te komen tot wat hij de verbonden tijd noemt, verschuift Wijnberg zijn aandacht naar behulpzaamheid als kracht achter de beschaving en ecologisme als noodzaak om uit de paradox te komen. Wat betreft de eerste pijler leunt zijn betoog op dat van Rutger Bregman ( [book:De meeste mensen deugen|45995328] ). Hoewel die auteur tegenwoordig ook op hoon kan rekenen, is Wijnbergs versie te relevant om opzij te schuiven. Integendeel, zijn beroep op collectieve voorspoed lijkt belangrijker dan ooit en vormt een welkome remedie tegen het denken in termen van geld (bbp, betaalde arbeid, rendement).
Vooruitgang is, kortom, niet alleen een kwestie van uitvindingen, ontdekkingen of een betere omgang met elkaar – vooruitgang is een verhaal dat we elkaar vertellen. Een verhaal over wat wezenlijk, werkelijk, wenselijk en waarachtig is. Een verhaal over hoe we onszelf, de wereld en de toekomst zien. Vooruitgang is een verbeelding om in te geloven.
Vanzelfsprekend is het boek eindeloos veel boeiender en complexer dan mijn weergave, die slechts bedoeld is voor mijn eigen begrip en reflectie. Wijnberg geeft tussen de hoofdstukken door overigens heldere overzichten om zijn betoog samen te vatten. Er zijn beslist journalisten en politici die begrepen hebben hoe wrang de huidige situatie is en dat een nieuw verhaal nodig is (dat verder reikt dan ‘laten we het nog één keer uitleggen’). Ik houd mijn ogen ervoor open.
Deze maand is het vijf jaar geleden dat samenlevingen massaal in lockdown gingen. Zoals velen herinner ik me die periode levendig, vooral de goede dingen. Met een zonnig appartement en een vast inkomen mocht ik van geluk spreken; ik was te jong om tot een risicogroep te behoren en te oud om gevoelig te zijn voor sociale impulsen. In Parijs las ik onder andere de dagelijkse bijdrage van Ilja Leonard Pfeijffer in NRC. De schrijver had in Genua niet alleen te maken met nog strengere regels, maar verkeerde ook in het land dat als eerste en het zwaarst werd getroffen in Europa. Vijf jaar na dato is het een plezier die bijdragen gebundeld terug te lezen. Ik meen dat Pfeijffer geslaagd is in zijn opzet om een ‘authentiek en eerlijk tijdsdocument’ te schetsen.
Als schrijver denk ik beroepshalve na over verhaallijnen, spanningsbogen en compositie. Ik besef dat dit dagboek om …
Deze maand is het vijf jaar geleden dat samenlevingen massaal in lockdown gingen. Zoals velen herinner ik me die periode levendig, vooral de goede dingen. Met een zonnig appartement en een vast inkomen mocht ik van geluk spreken; ik was te jong om tot een risicogroep te behoren en te oud om gevoelig te zijn voor sociale impulsen. In Parijs las ik onder andere de dagelijkse bijdrage van Ilja Leonard Pfeijffer in NRC. De schrijver had in Genua niet alleen te maken met nog strengere regels, maar verkeerde ook in het land dat als eerste en het zwaarst werd getroffen in Europa. Vijf jaar na dato is het een plezier die bijdragen gebundeld terug te lezen. Ik meen dat Pfeijffer geslaagd is in zijn opzet om een ‘authentiek en eerlijk tijdsdocument’ te schetsen.
Als schrijver denk ik beroepshalve na over verhaallijnen, spanningsbogen en compositie. Ik besef dat dit dagboek om literaire redenen een omslagpunt nodig heeft. Alleen al uit compositorisch oogpunt zou het wenselijk zijn dat ik zou kunnen schrijven over dalende grafieken, hoop, de overwinning van de haast vergeten normaliteit op de stilte en leven dat eindelijk herademt. Maar de werkelijkheid voegt zich vooralsnog niet naar mijn literaire behoeften.
Adrenaline, obsessie en saamhorigheid In de korte bijdragen komen alle onderwerpen terug die destijds relevant waren. Het aanvankelijke ongeloof, gevolgd door gruwelijke verslagen uit ziekenhuizen, waar artsen moesten bepalen wie recht had op een plek aan het beademingsapparaat. De overheidsmaatregelen: de lockdown (in Frankrijk: confinement), verlaten straten, de verplichte anderhalve meter, provisorische aanpassingen (plexiglas) in supermarkten en postkantoren, de opkomst van het mondkapje en later de gefaseerde afbouw. De financiële zorgen van velen, het verdriet om de dood van dierbaren die men niet mocht zien vanwege het besmettingsgevaar. Maar ook de veranderende dagelijkse patronen, het steunen van buurtwinkels, het straatapplaus (dat in mijn eigen straat nog tot de zomer doorging en een ritmisch ritueel werd), de adrenaline van ‘illegaal’ langer op straat zijn om een geliefde of naaste te bezoeken. De obsessie met cijfers en het gevoel van controle, de barre prognoses voor de wereldeconomie. En uiteindelijk ook de virusmoeheid en de manifestaties.
Is er iets veranderd? Janus knikt en schudt zijn hoofd. Zoals na de komst van de zwarte dood in 1347 en zoals na de grote pest van 1656 en 1657 is de stad onherkenbaar zichzelf gebleven en onwrikbaar herschapen.
Grote beloften voor de toekomst Pfeijffer nam de moeilijke taak op zich om verslag te doen van een periode waarin hij feitelijk niks meemaakt. Zijn stijl is soms wat gekunsteld of overdreven, maar het is hem vergeven. De vraag die na het lezen van de bundel blijft hangen, is wat we uiteindelijk van de pandemie hebben overgehouden. Destijds werden er grote beloften gedaan: minder auto- en vliegverkeer, meer ruimte voor de natuur, meer solidariteit met cruciale beroepen (verplegers, leraren). Behalve de toename van digitalisering en thuiswerken is daarvan weinig terechtgekomen. Op persoonlijk vlak kijk ik echter tevreden terug: als lezer heb ik me sinds de pandemie snel ontwikkeld.
Zes jaar na Congo schreef David van Reybrouck (1971) opnieuw een verhaal dat is georiënteerd op het Afrikaanse continent en gebaseerd op gesprekken die de schrijver voerde met mensen die het hebben meegemaakt, ditmaal de Belgische deelname (december 1992 - december 1993) aan een militaire vredesmissie in Somalië. Para beslaat echter nog geen honderd pagina’s en is geschreven in de vorm van een theatermonoloog.
Gruwelijke geschiedenis Aan het woord is een voormalige paracommando die een lezing geeft over zijn ervaring. Gaandeweg verliest de spreker zich in zijn verhaal, dat naar het einde toe fragmentarisch en onsamenhangend is. Het relaas roept vragen op over het mentale welzijn van de oud-soldaat, maar ook over de missie zelf: is het niet merkwaardig om een groep mannen uit hun omgeving te plukken en in een gebied te droppen dat hen volstrekt vreemd is en een gruwelijke geschiedenis kent? Kun je daarop voorbereid zijn?
Bij …
Zes jaar na Congo schreef David van Reybrouck (1971) opnieuw een verhaal dat is georiënteerd op het Afrikaanse continent en gebaseerd op gesprekken die de schrijver voerde met mensen die het hebben meegemaakt, ditmaal de Belgische deelname (december 1992 - december 1993) aan een militaire vredesmissie in Somalië. Para beslaat echter nog geen honderd pagina’s en is geschreven in de vorm van een theatermonoloog.
Gruwelijke geschiedenis Aan het woord is een voormalige paracommando die een lezing geeft over zijn ervaring. Gaandeweg verliest de spreker zich in zijn verhaal, dat naar het einde toe fragmentarisch en onsamenhangend is. Het relaas roept vragen op over het mentale welzijn van de oud-soldaat, maar ook over de missie zelf: is het niet merkwaardig om een groep mannen uit hun omgeving te plukken en in een gebied te droppen dat hen volstrekt vreemd is en een gruwelijke geschiedenis kent? Kun je daarop voorbereid zijn?
Bij de beelden van de soldaten in Somalië moest ik geregeld denken aan de stereotypen die Dipo Faloyin beschrijft in Afrika is geen land. Doordat Van Reybrouck onder de huid van zijn personages kruipt, blijft hij toch geloofwaardig. Ik vond zijn taalgebruik aanvankelijk wat opgeschoten en flauw-grappig, maar naar het einde toe krijgt het een poëtischer karakter. Ondanks dat stijl en vorm niet per se aan mij waren besteed, ben ik toch onder de indruk van zijn werk.
Op een keer mocht ik met de helikopter meevliegen. ’t Was regenseizoen. Die plassen daar, dat zijn hele meren! De pistes zijn weg, ge hebt niks van oriëntatie. En vanuit de lucht zag ik in die watervlakte een manneke van een jaar of zeven of acht op stap met een kudde van wel dertig dromedarissen. En die vond daar zijn weg naar een dorp, kilometers verderop, zo zonder niks. En die had misschien alleen een potteke kamelenmelk bij zich. Of een potteke yoghurt. Hij zwaaide naar ons met zijn wandelstok. ’t Is voor dat manneke dat we ’t deden.
In a way, Paradise by Abdulrazak Gurnah (1948) serves as a counterpart to Chinua Achebe’s Things Fall Apart. Whereas Achebe recounts the story of a West African tribe centred on agrarian culture encountering British colonialism, Gurnah’s novel explores East African communities centred on trade encountering German colonialists. The author makes the complexity and variety of late-19th-century cultural and political relations in present-day Tanzania tangible. By choosing the perspective of a twelve-year-old boy, he invites the reader to wonder along with him. Like his protagonist Yusuf, it took me some time to realise that ‘Uncle' Aziz is, in fact, something of a ‘Godfather’ figure, exploiting his ‘clients’ and taking their children as collateral. Gurnah’s world is a bitter one, where human life has little value, children become adults at a young age, and violence is part of everyday life. What struck me most is how Yusuf – and with him, …
In a way, Paradise by Abdulrazak Gurnah (1948) serves as a counterpart to Chinua Achebe’s Things Fall Apart. Whereas Achebe recounts the story of a West African tribe centred on agrarian culture encountering British colonialism, Gurnah’s novel explores East African communities centred on trade encountering German colonialists. The author makes the complexity and variety of late-19th-century cultural and political relations in present-day Tanzania tangible. By choosing the perspective of a twelve-year-old boy, he invites the reader to wonder along with him. Like his protagonist Yusuf, it took me some time to realise that ‘Uncle' Aziz is, in fact, something of a ‘Godfather’ figure, exploiting his ‘clients’ and taking their children as collateral. Gurnah’s world is a bitter one, where human life has little value, children become adults at a young age, and violence is part of everyday life. What struck me most is how Yusuf – and with him, thousands of others – has no say in decisions affecting him and even finds equanimity in his life of captivity. I will gladly read more of this Nobel Prize winner’s work in the future.
With a restraint that barely conceals the ferocity of his characters' passions, one of Japan's great postwar novelists tells the …
With a restraint that barely conceals the ferocity of his characters' passions, one of Japan's great postwar novelists tells the …
Wie het nieuws leest, de discussies op sociale media volgt en de debatten in de politiek bekijkt, kan haast niet …